Dr. Ernest Chan from PREDICTNOW #003
On our third episode of Fintech Underground by Alpaca we interviewed Dr. Ernest Chan, the Founder of PredictNow.ai and the author of multiple books.
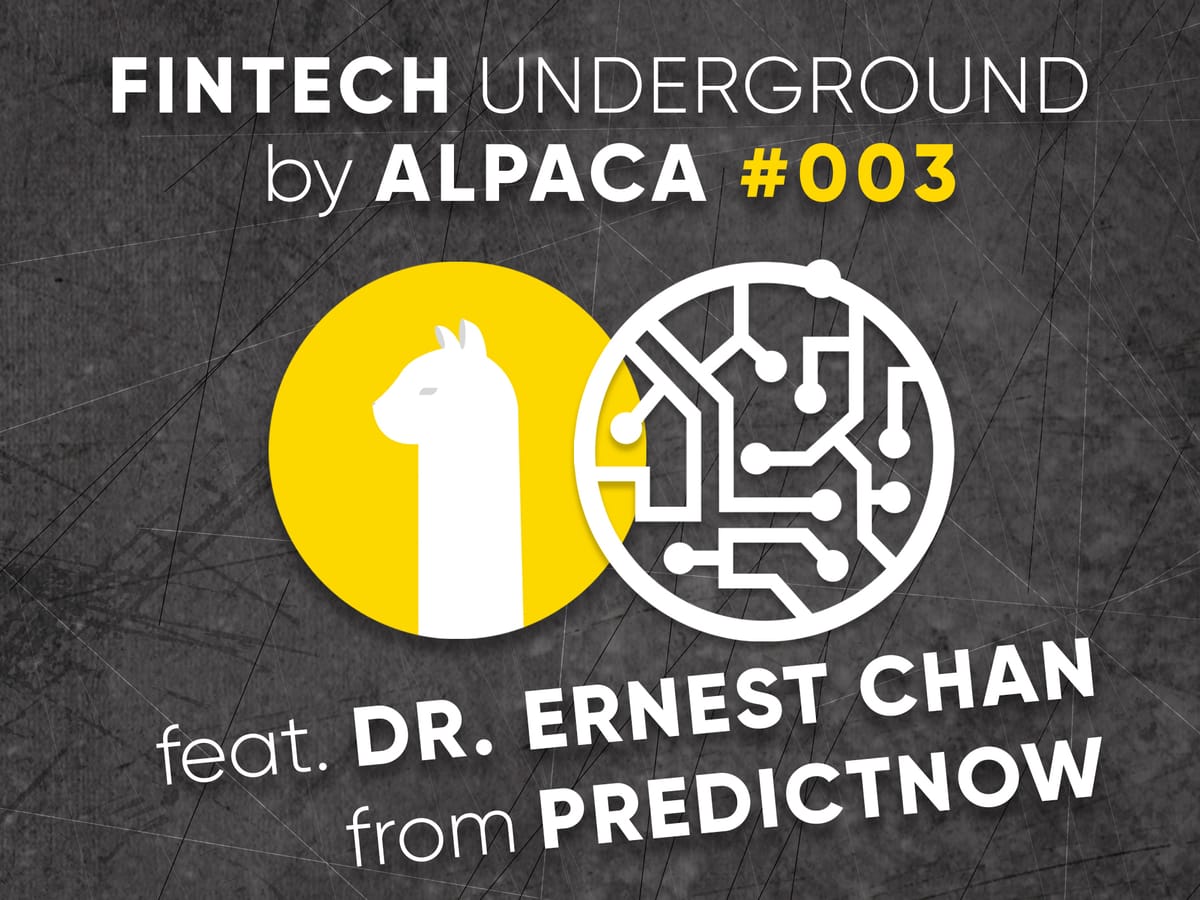
Any opinions expressed are opinions of the host and their guests. Alpaca Securities LLC does not recommend any specific investments or investment strategies.
Fintech Underground by Alpaca is a podcast devoted to all topics related to stock trading and APIs. From trading with algorithms or connecting apps or building out services, we aim to bring light to the different corners of Fintech.
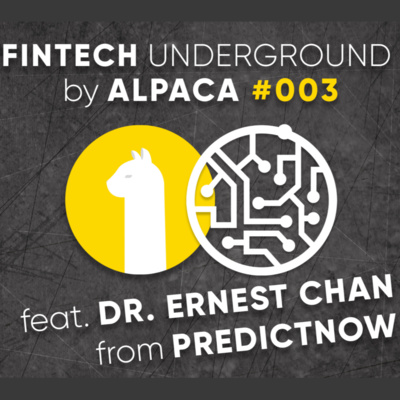
TL;DR
On our third episode of Fintech Underground by Alpaca we interviewed Dr. Ernest Chan. As the Founder of PredictNow.ai, along with the author of multiple books on quantitative and algorithmic trading, Ernest discusses his experience at Morgan Stanley, the hedge fund industry, and his thoughts on the recent surge in retail trading.

Summary
As a prominent figure in the quantitative and algorithmic trading space, Dr. Ernest Chan has had quite a remarkable career. Starting off in the machine learning department at IBM, Ernest worked on developing trading strategies using AI and neural networks. Many people might have first been introduced to algorithmic Trading through his popular books Quantitative Trading: How to Build Your Own Algorithmic Trading Business and Algorithmic Trading: Winning Strategies and Their Rationale. However, his contributions to the fields do not stop there, as he founded PredictNow.ai, allowing people to apply machine learning predictions to their investment data without any prior programming knowledge.
Find the full transcript below. To hear more CEO/Founders of the most interesting Fintech companies speak about their experience in the industry check out our other episodes below:
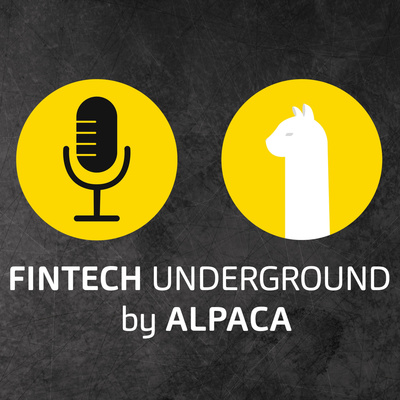
Full Transcript
Jason: Welcome to FinTech Underground by Alpaca a podcast devoted to stock trading API from trading, with algorithms to connecting apps, to building out services. Alpaca is built for developers and traders. And with that being said, let's get started. Hello everybody. Welcome back to FinTech underground by Alpaca.
[00:00:17] In each episode, we aim to explore a different area within FinTech. I really cannot wait to start off today's episode, featuring Ernie Chan, the founder of Predictnow.ai. Additionally the author of multiple books on quantitative and algorithmic trading. As usual, I would like to introduce our hosts, Yoshi CEO, and co-founder of alpaca.
[00:00:36] As much as the community would agree. Ernie's books have been a great resource for algorithmic and quantitative traders of all levels. So let's get started.
[00:00:45] Yoshi: Hi, Ernie, welcome to the podcast and it's always nice to see you here.
[00:00:49] Ernie Chan: Thanks for inviting me, Yoshi. Good to see you too!
[00:00:51] Yoshi: I think like we definitely want to get started off by really introducing you from your world because it's like, you know, I think you know, a lot of people know about you from your algo trading books and obviously a lot of you know seminars, podcasts, and interviews from Quantopian.
[00:01:07] So the, a lot of you know, algo trading related places, but I think I see you as the cool hedge fund person, Quant Finance professional person, but like, you know, could you please explain what you do from your world instead of mine? Okay.
[00:01:21] Ernie Chan: All right. Actually, before I started in the you know, Hedge fund industry.
[00:01:26] I was a machine learning researcher at IBM, TJ Watson, and it was a very cool place. So I guess that's how I pick up the coolness.
[00:01:36] Yoshi: That's right. That's right. Yeah. Yeah.
[00:01:38] Ernie Chan: So I pick up some you know, some techniques that I learned from IBM, you know, we, we had a very interesting group, as many people know the group had produced some of the top hedge fund managers in the world, like Bob Mercer.
[00:01:50]And Peter Brown and so forth and you know, who are running Renaissance technologies at a different point in time. So I learned a lot from these people and you know, I ended up also in the finance industry, but you know, I am a sort of a city person at that time, maybe not now, but so I decided to go to work in New York in Manhattan, instead Yeah, going elsewhere in the suburbs. So you know, it, and it's interesting that I actually did not find machine learning to be very helpful to me until recently, because. A lot of times, for the longest period of time, people couldn't figure out how to use machine learning effectively in finance, because the issue of overfitting and data mining is ever-present, you know, when you have a, it's so easy to generate great backtest using machine learning.
[00:02:40] But whenever you trade live, it's everything falls apart because it's really overfitted to noise.
[00:02:45] Yoshi: A lot of AI and machine learning. So like, you know, you worked at many wall street firms and those so like millennium, you know, hedge funds. So you weren't really necessarily using AI and machine learning at that time.
[00:02:57] Ernie Chan: Then we tried you know, nobody can accuse us of not trying so everywhere I go, people say, Hey, you know, I should not even try it. In fact, that's my first job at Morgan Stanley was joining their data mining and AI group. Right. And one of the mandates of that group, not the only one, but one of them is to develop trading strategies using AI.
[00:03:19] And I heard they were some success from other you know, formal manager, but when we. I myself tried it, with my you know, contemporary teammates. The success is purely in backtests. So you know, we, we try to apply again live in Credit Suisse, in a prop trading group in Credit Suisse. Again, the research is much more impressive than the production than the deployment
[00:03:44] Yoshi: Interesting, is that like, at that time you were already like, you know, using.
[00:03:49] Like those knee-deep neural nets around, that came out again popular around 2014, 15. Or like you were doing a different type of machine learning AI method back then?
[00:04:00] Ernie Chan: Deep Learning wasn't invented yet when I joined Monsanto and Credit Suisse so we were using neural networks and random forests, but actually not necessarily random forests.
[00:04:10] We use a classification and regression tree. I don't think that even when random forest, the notion of ensemble learning was that popular then, I mean, it's invented. By already, but it is not, you know, really as popular as it is today, you know, so now with deep learning and all this ensemble, learning methods, being popularized, everybody knows that the way to reduce out of sample errors, the technical term is variance to average over many models and introduce as much randomness during training as possible.
[00:04:42] But at that time, well, we were in the early stage of applying machine learning to finance. We didn't know that when we see a great backtest, we said we were so excited. So that we didn't think about the tension between Path bias and variance, the error in the training set versus the error in the test set that was not top of mind for us.
[00:05:01] And as a result, it wasn't particularly successful during those years.
[00:05:05] Yoshi: You think like, you know, those deep neural nets. Were there back then, did you think the results were different or this like, you know, approach to AI? Just back then? Wasn't something that like around the time it just wasn't working.
[00:05:18] Ernie Chan: I think that you know, we had the deep learning technique, it might improve the out of sample result, but I still am skeptical. And you know, I have certainly read many academic papers recently that claim, you know, deep learning has done this and that in finance. And some of that might even be true because I, you know, you can hear a certain degree of sarcasm in my tone is because a lot of times when you try to replicate what an academic research claim works, I would say that at least 50% of the times you can't replicate it.
[00:05:51] It is a big problem in financial research. And most of the time, no one bothers to replicate it because if they were successful, they won't tell you and if they failed, they wouldn't bother to tell you. So it's unlike medical research, right? You don't, if you have developed a new method of you know, curing the covid 19, that will be like 100 groups in the world trying to replicate it and try to publish a paper within two months.
[00:06:14] But in finance, you know, you publish something. The people who were successful in replicating it, won't tell you. Certainly to keep it completely quiet. And the people who fail to replicate it also won't tell you because why bother maybe reflect poorly on themselves?
[00:06:28] Yoshi: So that's like a pretty, like, not cool environment, but like, you know, I understand it's like, you know, kind of zero-sum in a way, like, you know, someone's buying than someone's selling you know, things happen.
[00:06:38] And like, and I actually wanted to save this question to the end, but like, because we were talking about like out of sample, And also this like industry as a whole, I wanted to ask you, like, a lot of people are thinking about GameStop stock, right? So like this AI model and out of sample in obviously like, you know, as the Robin hood Vlad was talking about you know, deposit, you know, shut it up because of the value at risk calculation of the, you know, stock fall, how did that impacted your AI and the model, or like any strategy that you were running and why do you think about that impact.
[00:07:10] Yeah, whole hedge fund industry. Around that time,
[00:07:13] Ernie Chan: They have many layers there's of interference. Let's just focus on one layer, which is the, you know, how AI deals a, it obviously AI have not had learned, you know, have not seen this kind of phenomenon before it's brand new. So therefore it won't be able to make a correct prediction out of the sample.
[00:07:30] But there's another issue which I have sort of recently is that if you included the last, you know like four or five months, craziness in your training data, it is also not optimal because I viewed that as a one-off phenomenon. You know, like January is completely different from February. The markets seem to have reverted to the old days and January was completely crazy.
[00:07:52] So if you had used those data as part of your training model, it might not be optimal. And that points to an issue with financial machine learning, is that a lot of people in machine learning say, Oh, the more data, the merrier, right. You know, who, who doesn't want data it is like, gold, you hear that often data is like gold, the more gold the better, but it's not true in finance because some of these data might come from a period, like the last four months that is completely exceptional.
[00:08:17] You won't see that again. So if you include that, as part of the training is actually produce a model that doesn't work in normal times, and that's not ideal,
[00:08:26]Yoshi: Like, you know, impact do you think that impact was by this you know, game stop you know, stock fiasco, and now this old Redditors meme stocks, like as a quants finance industry as a whole, what did you think that impact was?
[00:08:40] Like, do you think, like impact was minimal because like, you know, probably most of the funds shut down the whole strategy because the market was crazy or like, you know, you think that a lot of people lost money or made money from running the strategies like automated strategies
[00:08:54] Ernie Chan: You know certainly the traditional. For example, statistical arbitrage strategy that short bad stocks and long, good stocks got a big hit on in January. You, you could see that, you know, Ren tech institution and equity fund were down. What 9%. And so forth, but I believe that the market has completely changed from January. So, so I'm sure that they have, will, they have recovering some of that money.
[00:09:20] And, you know, I see from our own trading that the market has really returned to what it was before this craze. I think the impact is temporary. Just like, you know, and we have seen this movie before we have seen this kind of Abnormal events, for example, during the dot com bubble, right? You know, even Joe sorrows or Warren buffet, actually, Warren buffet made wrong bets during the bubble because they thought that things couldn't get that high for a company that has negative earnings for billions of dollars.
[00:09:49] And then he was proven right after the.com bubble crashed for burst. Right. And so, and, and, and again, in 2007, in the summer, we had a quant fund meltdown and again, ren tech was hit and, you know, all this long, short equity fund was hit, but again, they recover in the rest of the month. They actually ended a year positively.
[00:10:10] I mean, if I remember correctly, now there are some groups that shut down. For example, Goldman Sachs I don't remember the name of that group global offer fund or something. It wasn't in my book anyways, And it was it, they shut down, you know because they didn't hang in there long enough. Right. As long as you hang in there long enough, you will eventually recover from this craziness because the craze is short-term.
[00:10:33] So for example, now people cannot just keep paying, you know, the stimulus check. Yes. Two trillion more might be coming, but you know, it's not endless. They don't have unlimited resources to buy a call option on GameStop and see them expire out of money. There is an end to it. And one interesting prediction that I have, and also amazingly bill Ackman has, you know, I was very proud to have the same opinions Bill Ackman and we're just that when the warm weather returns and when the vaccine rollout, become much more prevalent, people are going to spend money on food on travels, on entertainment. They want to get out there. Party, eat. Well, other than sit at, stop, sit at home, and trade game stop. Right. You know, this is not a life in what kind of life it is to sit home every day and trade GameStop.
[00:11:23] So go out there more. And at that time, the market will be more, much more normal. The same sell-in may and go away might actually be true. This, when may come around Memorial day weekend in the U S. People, started to travel, go out to swim. Well, get some sunshine. And the craziness would subside.
[00:11:42] It is my prediction. And also amazingly Bill's Bill Ackman.
[00:11:47] Yoshi: So, because I think like there's also this you know, opinion where this is just the beginning because you know, now a lot of people has basically access to Robin hood app and now. You know, public and you know, WeBull and lots of others.
[00:12:03] Or do you see that from your experience? This is like, Oh, you know, I've seen this before. Like a lot of people get around and try to do stuff, but like at the end of the day, it's, you know, coming back to the fundamentals, is that what you see?
[00:12:15] Ernie Chan: Or, well, I, I think that it will. Push trading retail trading to a high level than before.
[00:12:21] So certainly there are a good percentage of these people who suddenly become stock traders. will remain stock traders. Now, I don't know how many percent, maybe 20%, maybe 25%. So we will certainly push retail participation to a higher level than before the pandemic. That pretty much, I think is a safe guess, but the volume may not be maintaining at the peak level. As we saw in January.
[00:12:46] Yoshi: No, thank you very much for all these like recent events stuff. Like this is always interesting to hear from a different type of players in that industry about this whole game stop stuff. But, you know, just going back to a little bit to you know, what you do, you know, obviously like, you know, you worked at big wall street, great firms and you know, hedge funds.
[00:13:03] And then, you know, you started your own firm, basically. How was the journey like, you know, when you decided to start your own thing and probably like fundraise yourself and why did you decide to do that? And like, you know what, like, you know, why you didn't stay at the big firm to do what you do
[00:13:20] Ernie Chan: So in a big firm there's great pressure to produce something new and complicated to impress your manager especially for quant groups. I mean, if you are a discretionary trader, maybe you don't have that pressure, but as a Quant. And the quant trader or quant researcher you have the pressure to say, Hey, I'm the smartest guy I'm smarter than this next guy who only has an MBA.
[00:13:41] Right. So, you know, I have a Ph.D. and this guy, only has an MBA, you know, so you need to produce something, Oh my goodness, deep learning, or, you know, whatever, fancy state-space model you know, wavelength analysis. And you know, all these fancy terms, you want to roll them out. So to impress people.
[00:13:57] To justify that the fact that, Hey, you know, you are contributing otherwise, you know, if you, all you trade is like a buy low sell high strategy. Even if you make money, say why do I need to hire you? Why any, any college students know how to buy or sell? Right. So, so, but the problem is that what I've found since is that the more complicated the model is, the more likely this is to overfit.
[00:14:19] To the past data and there's very little signal and a lot of noise. So actually simple strategies tend to actually work better. In fact, a lot of MBA is doing better than Ph.D., a lot of college students better than MBA. That's what I realized. And I say, well, I can't do that in. When I'm working for someone, because if I switched to a strategy like that, they, you know, they will not be impressed.
[00:14:44] So I have to quit and start to trade for my own using the simple strategy and it proves to work. And of course it cannot work forever, you know, buy low, sell high. Yes. It's might work for a while, but it's not going to work forever because everybody figures it out sooner or later. So yes, after you started to work, you might have to add bells and whistles it.
[00:15:02] So. Buy low sell high yes. But when do you buy, okay, that's the condition? You know, what, under what condition you should not buy. And that's something that I have lately find out, you know, in the last year or two found out machine learning can actually help a lot. So basically the basic strategy should be simple, buy low, sell high.
[00:15:20] Alright, no problem. But machine learning can tell you when not to trade. It doesn't, shouldn't tell you when to trade, but this should tell you, Hey, currently the condition is not so great for buy low sell high so it's, don't trade it today. That's where machine learning can help. So this is, I find most successful is a combination of simple, basic strategies that you can explain to someone in a few sentence and a fairly sophisticated. Machine learning risk management layer that you impose on top of it. That seems to be the best combination.
[00:15:52] Yoshi: That sounds like a, you know, same as a regular life lesson. Make sure you learn to say no to the things that you shouldn't do. That's actually the same as a life lesson. But so, and those, I think like, you know, among the listeners, I'm sure that, you know, they're you know, a lot of people who's you know, Oh yeah, my algos doing well you know, my strategies are good.
[00:16:10] Like I'm super smart. How well do you need to be to start your own hedge fund? I mean, like, I think you're doing this for. A little bit more than 10 years, right by yourself. How well do you need to be to do that? Like if you were to tell to those people who want to start a hedge fund?
[00:16:26] Ernie Chan: Well, certainly, you know, consistency, you know, a lot of people think that, Oh, I need to the high return.
[00:16:31] I need whatever 50% some guy told me, Oh, I want 1% a day return from your fund to forget about it. If you might trade Bitcoins, you might get that. No, I think consistency is the most important for a new hedge fund. You know, it's not so much return, you know, even if you get like, Oh 10%, 9% return, if you can get that every year, whether the market crash or market go up or down, You will get millions and millions of assets is not really returns that many hedge fund investors are looking for.
[00:17:01] Although, of course, it shouldn't be negative, it is really consistent. A lot of hedge fund investors are very wealthy already. They don't need an extra 10% return, but they want to make sure that the money is generating yield. That is better than what they can get from buying bonds. And yet the risks are not high, so they don't have a crash risk.
[00:17:24] So, yeah, consistency and a solid risk management system is. Paramount for being a successful launch of a hedge fund. Yeah. The high return might attract some retail investors that are chasing the 1% a day return, but sophisticated high net worth individuals know not to chase that kind of moonshot.
[00:17:45] Yoshi: That's super important, right. Because I think that, you know, hedge fund the investors already rich. So like, you know, they're just looking for consistency and I think it comes back to, you know, making sure that you don't do the choices that you should do and making sure that like, you know, you try to be consistent.
[00:18:03] And, but yet, like, I think a lot of people, like, I think individuals in the retail investors, of course, they look at the performance of the things instead of, I guess, like, you know, Sharpe ratio and the volatility. Over the performance, which I think like, you know, that Sharpe ratio itself. Like, you know, how many people would understand this, you know, in terms of the concept.
[00:18:23] And I think like, you know, the people who were using Quantopian and they actually building the models I think like, you know, the, it was really good to educating that, but like, it's a really hard concept. Like, what is it that? Consistency. And like, you know, of course looking at the game stop it's like, yeah, I made a lot of money.
[00:18:37] Like, you know, doing this, like what, like $40,000 in a, like two days, you know, it's, that's why it goes viral.
[00:18:44] Ernie Chan: That that's right. You know, they are actually a deep mathematical result that tells points and I've written it. Many times on my blog and actually in the new edition of my first book that we are publishing.
[00:18:56] So it's very easy to understand. Right? So let's say you have a, you bought a stock and the first a hundred percent, and then the next day it dropped 100%. Well, if you compound a to return, it is not that many people thought, Oh if it goes up 100%, one day and then goes down 100% the next day you're back to nothing.
[00:19:15] Right. So nothing happened. But that's not true. If you go up 100% of the day and go down 100%, the next day you have zero equity, you lost them all. So you can see from there it's an extreme example, that risk is very bad for long-term compounded return. Right. So even if every day you can go up X percent and the next day you go down 6% and then up X percent and then down X percent, you might think that okay.
[00:19:39] You know, at worst what's a big deal. I'm flat. No, you're not flat because of the volatility. You can actually keep going down because of that compounding effect. So the risk is really bad for long-term growth.
[00:19:52] Yoshi: Right. Right. I mean, I mean, it's great to really emphasize that point because I think a lot of people.
[00:19:57] Misunderstand the importance of it of the, you know, consistency. Thank you very much for this. I think like, I want to really even go back to, you know, your era, but even before finance, but I think like, you know, it's not, I don't know if it's unique or not, but like I think there are a lot of people going back and forth between this tech, like deep tech and AI and the deep learning and to you know, finance field as well.
[00:20:19]You know, there are people who go into finance. And goes into the tech after that. And there are people going into the tech and then goes into the finance. And I feel like, you know they're like, you know, constant, I think that you know, people going back and forth by your case, you went into.
[00:20:34] Basically tech first very much technical technology. And then you moved to finance. And I think like, you know, there's, of course, different context, as you've been talking about differences between the finance and medical industry about a research paper and they, you know, talking about over-fitting in the finance industry.
[00:20:51] So I think that needs a, some different unique lens to that, but. Do you think that, and I always ask this to the people who I'm talking to. Is this like, you know, I wouldn't say like just a FinTech, but like he's a finance industry worth it. That you moved, you made a jump big jump from you know, IBM like researching on AI to this, you know, hedge funds, you know, managing money, like, you know, is it worth it?
[00:21:16] Ernie Chan: It's really a very you know, individual, you know, specific to the individual. So I would say that you, you should only make that move if you are already passionate about finance because finance is an Island. Once you get into finance, it's very hard to, a lot of techniques are, may not be applicable.
[00:21:34]To the outside world, we have some exceptions. We have actually reasonably found you know, in my new startup project. Now we have found a technique that would make trading strategies, construction much more effective, which is also applicable. We think too many industries in the optimization field, for example, but that's rare most of the time innovation finance stays in finance and it's not useful for anything else.
[00:21:58] And so you would only really want to switch into finance if you are really passionate about it. And the rewards of course are great, not just, you know, financial-wise, but also in terms of an intellectual challenge because finance is a very quantitative field, you know, textbooks run to hundreds of pages with integrals.
[00:22:16] You can read a finance book that has nothing but integrals on page one to page 350. So, so it can be very intellectually challenging and very rewarding in that way, as well as financially. But. It is difficult to jump out of it once you're in it, because you you're just such a specialized area.
Yoshi: That's super interesting because you know, while we are building out Alpaca you know, it's not just a tech company, it's a, you know, a lot of regulation, a lot of compliance that, you know, we have to really care about.
[00:22:47] And, you know, I think like, you know, that's pretty different. If we're not in the finance. So like, you know, I appreciate your view on that. And you know finally, like, you know, do you want to like, you know, touch a little bit on the predict.ai, you know, you started it, I think alongside with you know, your hedge fund business.
[00:23:03] Do you want to give, like, you know kind of few sentences pitch, what you do on you know, predict.ai?
Ernie Chan: [00:23:09] Sure. So, as I said earlier, the best usage of machine learning we found is, as you said, Risk management is to filter or trade rather than the suggested new trade. To filter a trade means that we need to compute the probability of whether a trade will be profitable or whether the decision will be profitable.
[00:23:27] Right. So we deployed that quite successfully in the last year or two. And so we thought that this might be useful to other traders people ask, why are you letting other people, other traders, unveiling our alpha. Well, it's not unveiling our alpha because it's purely a risk management system. We are not telling you what to trade.
[00:23:44] We are telling you what, when not to trade. So so we spin that out as a FinTech company called predictnow.ai. So people can use it without any code. The other issue with machine learning is that even though there's a lot of open-source software out there, psychic learn like GBM, TensorFlow, If, unless you are really a professional data scientist, it is very, very time-consuming to put them together.
[00:24:08] Especially if you have no data to start with, you need to get the data. Data is 80% of the time and software is 20% of the time. But even for that 20%, unless you are an expert, it is a tremendous hassle to put together a pipeline for machine learning. So that's why we put together this online system predictnot.AI where you can access it without any code is a no-code interface.
[00:24:31] If you want, you can also access this through our API. And actually, you know, I think we had to develop a connector with the alpaca accounts so that you know, the alpaca users can use it through our API.
[00:24:43] Yoshi: Well, I appreciate you explaining that and you know, thank you very much for coming to the show and thank you very much for sharing your experience, please you know, give the very quick upload that we can find Ernie with a lot of books and, you know, interviews and of course now predictnow.AI, you know, thank you, Ernie.
[00:24:59] Ernie Chan: Thank you, Yoshi. Thanks for inviting me.
[00:25:01] Jason: I also wanted to thank all of our listeners for joining us today. On this episode of FinTech underground by alpaca is always check out all of our past episodes on all major streaming platforms podcasts can be found Thank you.
If you liked this episode of Fintech Underground by Alpaca make sure to check out our other episodes below:
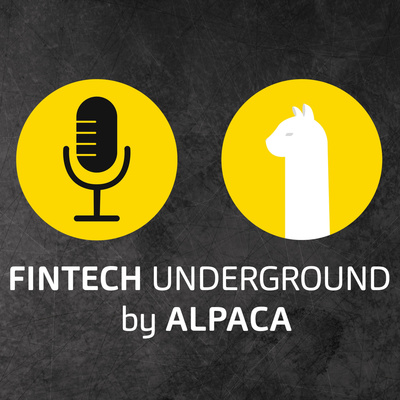
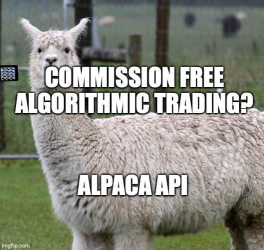
You can also follow Alpaca and our weekly updates on our LinkedIn and @AlpacaHQ on Twitter!
Brokerage services are provided by Alpaca Securities LLC ("Alpaca"), member FINRA/SIPC, a wholly-owned subsidiary of AlpacaDB, Inc. Technology and services are offered by AlpacaDB, Inc.