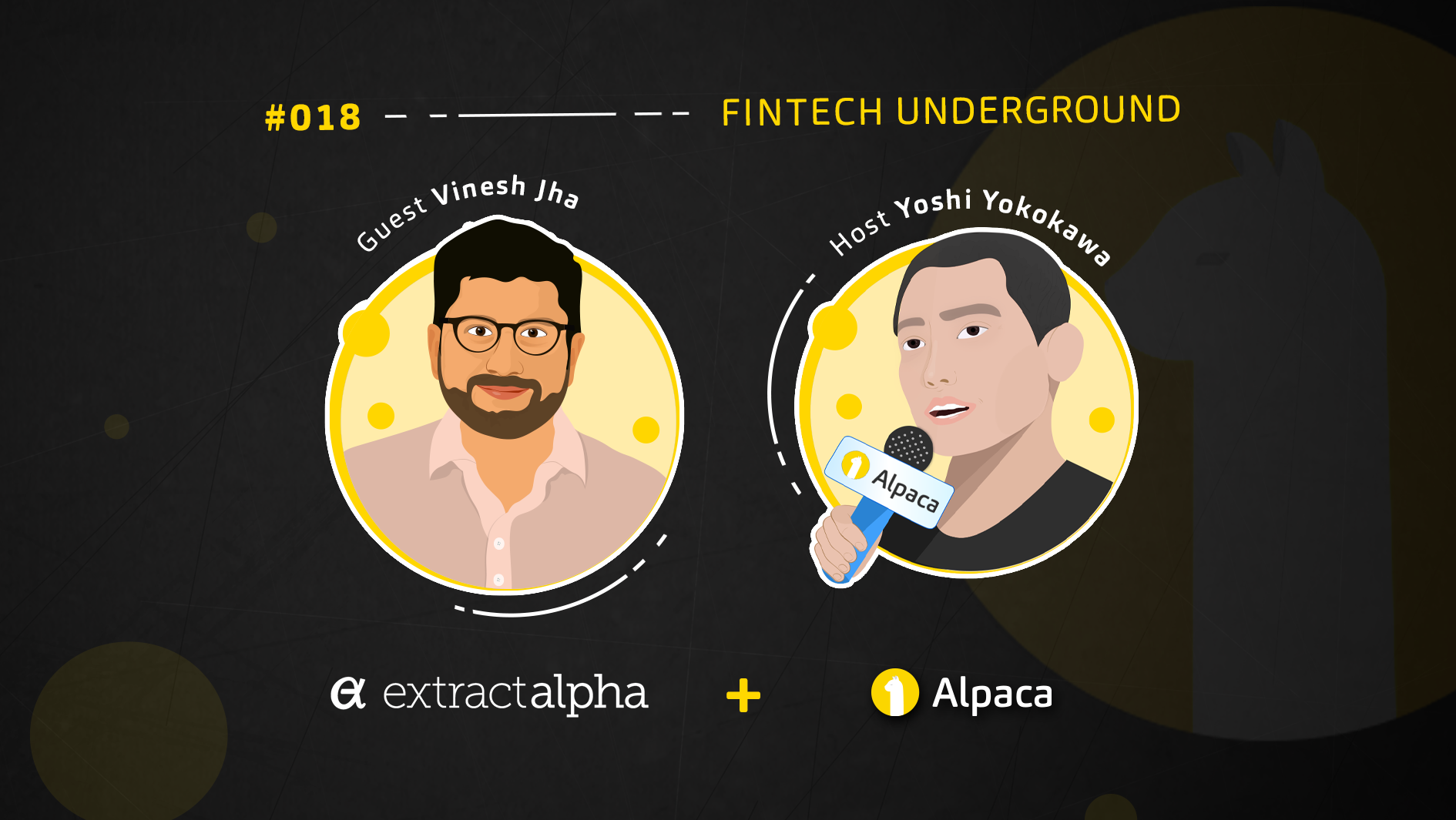
Any opinions expressed are opinions of the host and their guests. The content is for general information only and is believed to be accurate and reliable as of posting date but may be subject to change. Alpaca Securities LLC does not recommend any specific investments or investment strategies. Alpaca Securities LLC does not provide investment, tax, or legal advice.
Fintech Underground by Alpaca is a podcast devoted to all topics related to stock trading and APIs. From trading with algorithms or connecting apps or building out services, we aim to bring light to the different corners of Fintech.
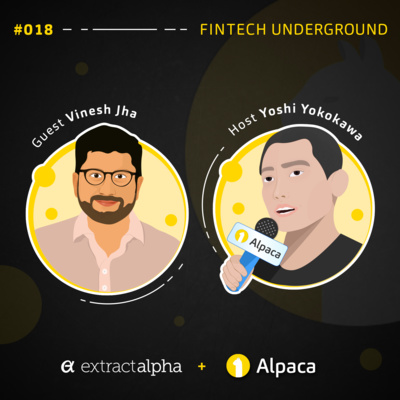
TL;DR
In Episode #18 of Fintech Underground by Alpaca, we interviewed Vinesh Jha. As the CEO of ExtractAlpha, an independent research firm dedicated to providing unique, actionable alpha signals to institutional investors, Vinesh discusses alternative datasets for investing, crowdsourcing financial intelligence, and more.
Full Transcript
Crystal [00:00:00] Hi everyone. Welcome back to Fintech Underground by Alpaca, the podcast devoted to stock trading API. From algorithmic trading to connecting apps to building out services, Alpaca is built for developers. In each episode, we explore a different area within FinTech. Today, Alpaca CEO, Yoshi Yokokawa is joined by Vinesh Jha, CEO of ExtractAlpha, an independent research firm dedicated to providing unique, actionable alpha signals to institutional investors. So let's get right into this educational episode.
Yoshi: [00:00:34] Thank you very much for coming to our podcast, Fintech Underground. How are you?
Vinesh: [00:00:38] Good. How are you doing Yoshi?
Yoshi: [00:00:39] Good, good. It’s been a while since we chatted, we were talking in the pre-Zoom era. It's been pretty wild, the world’s shifted since then.
Vinesh: [00:00:50] Definitely, yeah. We spoke in 2016, introduced by mutual friends, so it's been a long time. And definitely a different world now.
Yoshi: [00:00:58] Yeah. And I think it was through Quantopian, and I think Quantopian has been bought by Robinhood and the world is moving in many places. But firstly, I'd love you to introduce ExtractAlpha and also yourself.
Vinesh: [00:01:16] Sure, yeah. So I guess I can start with myself. I'm a quant guy. I started my career on the sell side, actually at Salomon Smith Barney back in, I think 1998. As a quant group there. About a year and a half after that joined a San Francisco-based startup called StarMine. That was in the analytics space, I guess today we'd called a FinTech. That term didn't exist then. Spent a bunch of years there building up their analytics. That company eventually got sold to Reuters and is now part of Refinitiv and the LSE Group. And that's been a bunch of years in prop trading in New York. So it was really building and training quantum models, quantity strategies, equity market, neutral ones globally. It's been a bit of time at Merrill Lynch. And then most of the time was on a prop trading desk at Morgan Stanley called PDT, process-driven trading. So I was there from 2007 to 2013. Around that time, PDT was spinning out of Morgan Stanley. They had to spin out and do the Volcker rule. So that's when banks couldn't hold prop trading groups.
Right after the spin-out, I left New York and I came to Hong Kong with no particular plan. I knew I didn't want to trade anymore and wanted to do something a little more entrepreneurial, I didn't know what. But I was thinking back on what I had noticed about data in the years I was trading and the clear thing that stuck out to me was thinking back to August 2007, and some listeners might know that was we've variously called the quant quake and the quant blow up. Essentially, what happened at that time was a lot of quantitative strategies had drawdowns all at the same time. So over three days, many of these strategies had huge drawdowns. It turns out they're much more correlated than anyone thought, to each other. And the reason for that, it turns out, was they were all kind of trading the same stuff.
They were trading the same types of instruments. They were trading based on similar types of data. And, you know, similar inputs give you similar outputs, give you similar strategies. So their holdings, if one person sells, everyone else's portfolio suffers. So the real problem was there was no differentiation in data. So that got me thinking that what you really needed to do is differentiate your data inputs, and that would help investors, especially institutional investors, diversify away from their competitors and have a real edge. So I started thinking about unique sources of data, and this was 2013. We didn't have the term alternative data yet, but I started looking into weird datasets. I just thought of it as this weird dataset.
Yoshi: [00:03:49] So what kind of weird data was your first? The weirdest data that you cracked open?
Vinesh: [00:03:54] Oh, that's a good question. I found a lot of weird ones over the years. I don't remember particularly what is the weirdest, but I certainly explored. In 2007, 2008, I started looking at unusual sources of information from filings data and legal proceedings and all kinds of web data, all kinds of exotic things, right? Anything that could tell me something about a company, and oftentimes you start looking at these interesting or weird datasets and nothing comes of them. It's really an exploratory, very researchy, fun topic. But most of the time weird data sets are just that; they're weird. They're not actually useful. So that's the idea behind ExtractAlpha, right? The alpha kind of came from that, kind of trying to find if weird could be useful too.
Yoshi: [00:04:38] That's a really interesting point. Like weird [datasets], most of them are not useful, but you're finding weird and useful data at ExtractAlpha?.
Vinesh: [00:04:47] That's the idea, yes. So we start out with weird and we tried to find useful, right? We spent a lot of time looking at datasets that don't turn out to be valuable. And the way we do that is we have a whole quantitative process. We try to be very rigorous about our analysis of these data sets and try to find, first of all, a lot of data cleaning and data analysis, just at the very superficial level, like how much data do we really have? You know, how far does it go back? How many stocks does it cover?
Are there holes in the data? If it's not already tagged to a ticker or some kind of identifier, can we create that mapping? Is it a point in time? Like, do we know if the data is accurately timestamped, so all of this sort of superficial stuff you really need to do first. And only then can you get to the meat of it, which is, is there something, can we create features out of this data that help us predict something? And that thing is not always stock returns, by the way. We do a lot of work on trying to figure out if data sets can be predictive of earnings or revenue growth, earning surprise, or more fundamental things.
Because that helps you tell a better story and really build intuition around a data set and why it should be informative. So we try to avoid anything that's too sort of data mining and just throwing things against the wall and seeing what sticks.
Yoshi: [00:06:03] I think these days new companies started doing that, especially these memes stocks. It gave that tension and there are a bunch of like, where's the social media and especially not only Twitter, but Reddit sentiments. Are those the things that you were looking at back then, or was it a different direction of alternative data that you were looking at?
Vinesh: [00:06:25] Yeah, I don't think meme stocks were as much of a thing. I mean, certainly, there have been. Apple's been a meme stock for a long time, for example. We were certainly looking at web data, online news, and various postings, various different things like that. Even back in those days, various forms of crowdsourcing. So I use the term "crowdsourcing" sort of loosely, like, is there intelligence in what people are saying online or doing online? And that continues to be an important source of alpha and intelligence for us, but not just for meme stocks, really for any kind of company with an online presence, that can be useful. And that's one of the things, one of the types of data of many that we do look at and we have been looking at for many years.
Yoshi: [00:07:07] Yeah, got it. Do you want to specifically talk about ExtractAlpha as a company and what services that you offer?
Vinesh: [00:07:14] Definitely. We are sort of a combination between a research firm and a data provider. We do both of those things. So as I mentioned, we look for all these interesting, you know, weird datasets. We do a lot of research to try to find out if they're predictive of something, whether that's returns or earning some other sorts of KPIs that investors care about.
And when we find value, we basically provision those data sets in a variety of different ways. And that could mean, you know, raw but tickerized data. It could be derived sort of trading signals and we're starting to build some visualization tools as well. So the primary consumers of these data sets, whether they're derived are systematic, hedge funds also, you know, trading desks, asset owners. Asset managers as well, but the primary use case is for a hedge fund to take in these datasets and use it in a systematic way as part of their trading strategy. And so we help them do that, and we try to get the datasets in as good a shape as we can for that particular use case since it's sort of data for quants by quants. And although we have plans certainly to expand beyond that quant market, this helps us make sure that the data sets are very rigorously vetted by all of these very sophisticated users. So that's our primary client base.
Yoshi: [00:08:24] I think what's interesting is that a lot of big hedge funds also have data teams, right? And so with that said, when you sell data and the research, what kind of hedge funds in terms of the size [are they], is it really small, super startup hedge funds or big ones like Citadel, Bridgewater? What kind of guys are you dealing with?
Vinesh: [00:08:47] Yeah, it's a great question. When we started out, we really thought it would be primarily the small funds, because in a sense, I mean, they don't ever want to say this, but they're outsourcing some of their research. Because they're doing research and they're taking the fruits of that research and ingesting it. So we thought that would primarily be interesting to the smaller funds; to startups, the spin-outs, and so on. And that was certainly true. But to our surprise, we found that some of the larger funds, without naming names, were also very interested in our datasets as well. Now, the things that they're interested in tend to be different, they are more likely to be interested in something that's either raw data or something that's if it is a drive signal, it's a very, very unique one that they can't get anywhere else. It's somewhat exclusive to us. Whereas smaller funds might be potentially more interested in something that is fully derived by us, even on top of a dataset they could otherwise get, because they're happy to leverage the resources and the research that we've done.
Yoshi: [00:09:42] That's interesting. So do retail, hyperactive traders use that as well, or is it always institutions who use that product that you offer?
Vinesh: [00:09:52] So it's all institutions at the moment. Yeah. I mean, it's, it's really, I mean, the price points for these things are not at, you know, where retail investors can really use them.
We certainly have a few family office / high net worth types who are looking at this, but your everyday retail investor? Typically the stuff is still a little bit out of reach. We certainly have thoughts about how to get it within reach or get a version of it within reach, but that would involve a partnership potentially with an online broker or something like that. We're not really in the B2C business. We would need an intermediary for that, most likely.
Yoshi: [00:10:24] Got it. I think what's interesting is that, and this is also how we got to know each other from, through Fawcett of Quantopian and how individuals also have access to those back-testing frameworks and also certain datasets. And now, as you say, in order to destroy this whole correlation to quant investing the data is the key. Is that possible for no institutional investors? Basically retail or big retail investors to somehow utilize the data that's supposed to be unique and weird and useful, and so do the right performance or how do you think about this democratization of weird, useful data distribution and usage?
Vinesh: [00:11:12] Yeah. And so that's a big topic. I think some of these alternative datasets are starting to get into the hands of individual investors, but we come at it from a slightly different angle in the sense that we're really looking for datasets that are likely to be helpful and useful to investors as a whole, many of the data sets that we come up with are perhaps not appropriate for all investor types. You know, some of them might be too fast-moving or might cover markets that maybe those investors don't care about. So I certainly think that many of these things could be useful in helping individuals improve their trading, but it's also, I think there's a big education component too. Many of them are not even using traditional data sets or traditional sources of intelligence in ways that are actually helping.
Whether that's news analytics or even the fundamentals, basic data like fundamentals that are just widely available. I think there's a big investor education component that has to come alongside these tools. You don't want to give someone a Ferrari if they don't know how to drive yet, right?
So I think there's a big part of that as well. And I think a lot of investor education has to come through a lot of these platforms, like great democratizing platforms Robinhood and so on, that have allowed individual investors to trade. I think the question is, do they know how to trade it?
You look at a lot of these forums, whether it's Wall Street Bets or even TikTok Finance, and some of the advice that's given there is just horrible, you know? So it took long enough for institutional investors get informed about how to be quantitative, how to be systematic, how to not make sort of basic behavioral mistakes. And I would love to see that get democratized too, not just access to information and data, but access to knowledge.
Yoshi: [00:12:52] I think knowledge is a very important point. And because you come from very big, well-equipped institutions like Merrill Lynch and Citigroup, and actually doing the prop trading, you know, I'm also from Lehman Brothers and a couple of years in Nomura as well. The framework is there, resources are there. The system is there. But when we spin out from these big firms, how did you equip yourself when you don't have those surrounding resources around you that you actually realize [you had] after leaving? Right? How was your experience of going through that transition?
Vinesh: [00:13:27] Very much trial by fire. Yeah, it's a great point. No one really equips you for running a company the first time, right? And that's what this was. The company is almost completely bootstrapped. We didn't take any VC funding or anything like that.
So the things you learn is that you've got to do everything, right? I'm a quant research nerd. My comfort zone is sitting in front of a computer in a room and writing code and doing research and analyzing data. But all of a sudden, I'm doing sales and business development, accounting, hiring people, all kinds of things. The things that you'd never think that you'd need to do when you come from this institutional background.
I've worked at startups before, and successful ones, but never ran one from the ground up, based entirely on self-funding and with no real help. And also doing it interestingly in Hong Kong, which, when I started this in 2013, there was no startup culture at all in Hong Kong. There's more now, but back then everyone wanted to work for big companies, you know, an HSBC, Standard Chartered, you know, there was no real startup culture.
It's grown a lot since then, in great ways. But there was no startup culture. There's also the time zone difference of being 12 or 13 hours ahead of New York. Most of our clients are, and we're sort of our business partners are as well. So there are definitely some challenges around that as well.
But we've grown our team here and it's at a much more comfortable state than it was back in 2013, 2014, where things were very, very nascent, just like any startup.
Yoshi: [00:15:01] Right. And also you mentioned an interesting point when you moved to Hong Kong in 2013, you said you really didn't want to do trading anymore. Why is that?
Vinesh: [00:15:11] I guess I was just a little burnt out from it. It's interesting. Trading is a great adrenaline rush. Even if it's systematic trading, I wasn't sitting there picking stocks or anything like that, but you have a book you're watching and it's actually watching globally, you know, across three different regions. So you never really stop. The day-to-day of it, just making sure your portfolio is what it needs to be and no news items are making it blow up. You know, you don't have a short position that's suddenly tripled in size because of businesses hitting the news in some way, or some long position goes under because it's, it's actually a shell company and people are realizing that. So things like that, very interesting things can happen, but that day-to-day wasn't what was exciting to me.
What was exciting to me was the research angle, again. And so that's really why I wanted this to become a research firm, a pure research firm. We do not trade. We provide intelligence and research to people who do trade, and that allows us to avoid the sort of, I don't want to call them distractions, but the day-to-day nuisances of running a portfolio or of raising capital.
It also allows us to do a lot less in the way of, more than we used to, but we don't have to do as much compliance as someone who's an asset manager does. We are not currently a regulated entity. We have plans to potentially become one, but at the moment we're not. And that allows us to save a lot of time on compliance issues and all of that as well.
So it's just a lot of the operational stuff we don't have to do. We can really focus on what we're good at, which is looking at data and finding insights from it and finding alpha in it, and helping people trade better based on interesting datasets.
Yoshi: [00:16:45] Yeah. And I think like, you know, the regulation is an interesting point, right? Because when you step into a certain point, it becomes investment advice, you know, recommending some kind of strategies that may go into the territory. How have you been managing that? This whole regulatory framework?
Vinesh: [00:17:02] Yeah. So we have to do more and more on the regulatory side, just from a compliance perspective with the funds we work with.
So many of the folks you mentioned, the bigger funds have data teams. A lot of what those data teams do is compliance and due diligence, questionnaires, and so on. We do a ton of those. So very often when we engage with a larger fund, we'll have to fill out a 20-page questionnaire. It tells us what's the provenance of the data, how do we collect it?
How do we contract with other people who might have data that we acquire? Could it potentially include PII, things like that? So we have to be very careful about that. We go through all those processes. We have to. Any data vendor has to at this point, but also we're very clear that we are, what we're providing currently is not investment advice.
It's data. You go and do with it what you like. That becomes interesting because it means if we do want to provide something that's a little bit more directional, directly actionable, then we might have to become regulated. So that's something we're looking into, is do we become an investment advisor? Not an asset manager, but an investment advisor.
And that would allow us to do things that are a little more concrete. So two examples of that would be we could create model portfolios for our clients. So not just "here's some data or some rankings on stocks", but really "your portfolio might want to look like this and you might want to run it this way."
And that would allow some partnerships that we don't currently have. And another use case would be, we could actually create trade ideas. So I've done a lot of work in the trade ideas and alpha capture space, and that's something we can certainly get involved in by providing, you know, this stock is a buy today.
Not just, we rank it highly relative to other stocks, but we think you should buy it today. We think you should short this other stock today. That's, you know, at least under Hong Kong law, that's, that's likely to be investment advice. So that might require some regulatory approvals, but that's something that we're looking into.
And I think it's a natural extension of taking everything we've done and sort of boiling down to this very sort of actionable thing that could help a variety of different types of investors, you know, whether it's these sophisticated institutions, all the way down.
Yoshi: [00:18:54] That makes sense. And you mentioned your love of research and dealing with the data and figuring things out. I think there are many professionals that include people who are actually working at big banks or even university students, they seek to do that. I think we see how Quantopian grew and also this hype Numer.ai was able to get. I think it's really kind of related to and connected to this mathematics data curiosity that could be proven by the market, which is really the superstar people are also dealing with. How can they get to the level where you are actually fighting and competing where big funds also appreciate what you are generating to actually extract the alpha.
Vinesh: [00:19:42] Yeah. So I guess I can answer that in a couple of ways. I mean, one is if you're a quant out there and you're trying to get some notice and trying to get into the field and so on, you have some of the tools out there. Quantopian was great. And there are others that, that exist now as well, Numerai as you mentioned, and Quantconnect and others that are these great tools for people to get started, maybe get noticed in the space. In a way, we face the same thing. We're a small company that's trying to get noticed by institutions, but we really do that by showing our work and saying, okay, this is what we've done. We try to be as transparent as we can about the quality of our research and the rigor of the research we provide.
So when we're dealing with an institutional investor, we're as transparent as we can be without giving away our IP. So we say, this is how we source data. This is how we researched it. These are our findings. Here's a 20-page paper we wrote on our process. Trying to be transparent, it's not just an algo that makes money.
I don't think that's something that's appealing to an institution because they really want to understand, you know, this is really a back and forth. It's not just, here's some data, here's an algo. Go trade it. It's a conversation. But what did we do? How did we get there? How did we come to these insights? And what did we try that didn't work? We try a lot of stuff that doesn't work. I mean, that's the nature of research. And so that's actually interesting to people. They'd want to know, oh, well, we're looking at all these datasets, you know, can you give us some guidance as to which ones might be useful or not? Because these guys are faced with hundreds of datasets at any given time and they. They need to know where to start. So even if you have a big data team in your big fund, like the ones you mentioned, it's still an overwhelming process to figure out even where to start, you know, with all these really cool sounding weird sounding but cool sounding datasets that might be in the press.
You know, maybe you read something in the wall street journal about satellite data. You want to figure out is that useful to your process? It sounds super cool. Is it going to help you? Well, maybe, maybe not, right? It's not obvious. And that takes work and that's a conversation.
Yoshi: [00:21:38] You mentioned getting your work out there. What is that? If you were to pick one destination that you really utilized or paid attention to when you were trying to get your work visible to the industry so that individuals who are wanting to be like you can follow your path?
Vinesh: [00:21:58] Yeah, I think if you're someone who is interested, who's got a dataset, let's say that's interesting. You want to get it out to the world. They're all resources now. I mean, first of all, you can come talk to us, we are always happy to talk. [laughs]
Yoshi: [00:22:10] Actually, that is the best one. I don't think we should talk further.
Vinesh: [00:22:14] There you go. Yeah. That's it done. Well, I mean, one of the great things that used to happen was conferences, right? It's a little harder now since everything's just Zoom, but there are all these companies that are doing online conferences about data, but also quants. And some of them are structured as sort of speed dating between data buyers and data sellers. Some of them are structured speed dating between allocators in quant, hedge funds, or startup funds. Someone's got a startup small funds. People who are allocating to quants. You know, some of those conferences are for that. So those are great as well. And then, you know, there are also more academic types of conferences. For a while, I was involved in a group called CQA Chicago quantitative Alliance, and they had a startup wing here in Hong Kong, actually called CQAsia, which was great. I mean, it was academics, you know, students could come and participate. Industry practitioners and those things are fantastic because it's really an intersection between academia, you know, some of the big banks occasionally sponsored them, research companies, data providers, and really just getting into the weeds and great ways for people to learn about this stuff as well. I hope we get to a stage where conferences can come back. I think they're great when they're not just purely commercial things, but they're also kind of educational as well.
Yoshi: [00:23:33] I agree. We need to really bring back the physical events somehow someday. And the other thing that I wanted to ask was you mentioned Quantopian, Numerai. It also has the kind of old similarity of crowdsourcing of potential alpha, right? If you want to cook it somehow or you want to use it individually? What do you think about the direction in general?
Vinesh: [00:24:00] Yeah. Well, I think crowdsourcing is super interesting as I mentioned earlier. And one of the things that happened earlier this year is we actually merged with a crowdsourcing platform called Estimize. So that's something that I think is pretty interesting. I've been involved in that company. Since early days, I've been an advisor to them since 2013 or 2014 or so. And it's a great platform for basically crowdsourced financial advice, essentially. So people can put in their earnings estimates on companies, KPIs about a company, how many iPhones they think apple is going to sell, macroeconomic indicators.
So I think there's a ton of value in crowdsourcing financial intelligence, as well as, you know, things like algos, like what you mentioned. I think growing that business is a big part of what we're doing as well, merging the companies that that was another sort of thing that has been a really interesting educational experience, you know, first in corporate action we did. And then in terms of what we want to do, I think crowdsourcing is one direction. I mentioned the investment advice. We're building out more of a marketplace as well, so there are all these cool signals that we've built or other people have built. And we think there should be a better way for people to analyze those signals.
So we've opened up a lot of our analytics and we're building a new platform called AlphaClub, which we'll be releasing soon, which we're really excited about, which will allow there to be sort of more of a marketplace for really actionable, useful, in some cases weird but very cool signals. And we're hopeful that that'll be a really great place for people to dig into how we look at data and be more rigorous in the way they analyze data sets that are perhaps more, more derived, you know, more like a buy, sell signal or ranking system, and help them sort of dip their toes into this stuff. If they're an institution that's looking to understand it better, or even high net worth or individuals could also check it out.
Yoshi: [00:25:46] Yeah. I mean, can we integrate our alpaca APA execution into the actionable signals and data, right? That's what I definitely want to do.
Vinesh: [00:25:58] Yeah, we should definitely talk about that.
Yoshi: [00:26:00] Anyway, thank you very much for this. And to wrap it up, I always ask this one question. We are in the FinTech space, as you touched [on], there is compliance and regulation that we have to be mindful about. And also it's an extremely, super competitive space.
You know, a lot of super-smart people out there. If you were in a different industry, there are things that you may not have to worry about, but you have to in FinTech. So is it worth it for you to be in FinTech?
Vinesh: [00:26:28] Great question. I mean, is it worth it? I've thought so. I love doing this stuff and I think one of the most interesting things is that you can really look at markets and try to understand people's behavior really in the aggregate.
I think that's one of the most interesting things about it. I mean, crosslinking touches on that, but generally quantitative research, which is our focus, is really about understanding what people do. In the aggregate, when they get into the market, what their incentives are and whether those people are investors, traders, or companies, what company management does have, it can understand what they're really doing. What analysts are doing. We spend a lot of time researching south side analysts and try to identify value in that data. And we have some, some products around that. I think it's super intellectually challenging, in a good way. I think if you're interested in data, if you're interested in sort of technology, interested in human behavior, this is a real intersection between those things.
And I think it's a great field. And if you can find a way to get compensated for it, all the better, right? But it's also just an academic field as well, which I think is something that people don't necessarily realize from outside of it. It's really cool stuff. It's not just robots. It's people building the robots to do interesting things.
Yoshi: [00:27:30] Wow. That's the deepest answer that I've ever got on this question and thank you very much for that answer. I really learned a lot from you, thank you very much for coming to our show and I hope you have a good day over there.
Vinesh: [00:27:47] Great have a great night, Yoshi. Thanks!
Crystal: [00:27:49] Thank you for joining us today on this episode of Fintech Underground by Alpaca. As always, check out all of our past episodes on Apple Podcast, Spotify, and other major streaming platforms.
If you liked this episode of Fintech Underground by Alpaca, make sure to check out our other episodes below!
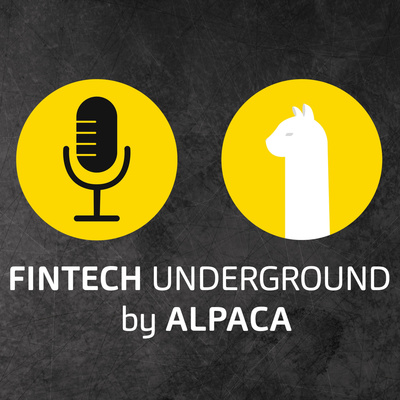
You can also follow Alpaca and our weekly updates on our LinkedIn and Twitter.
Brokerage services are provided by Alpaca Securities LLC ("Alpaca"), member FINRA/SIPC, a wholly-owned subsidiary of AlpacaDB, Inc. Technology and services are offered by AlpacaDB, Inc.